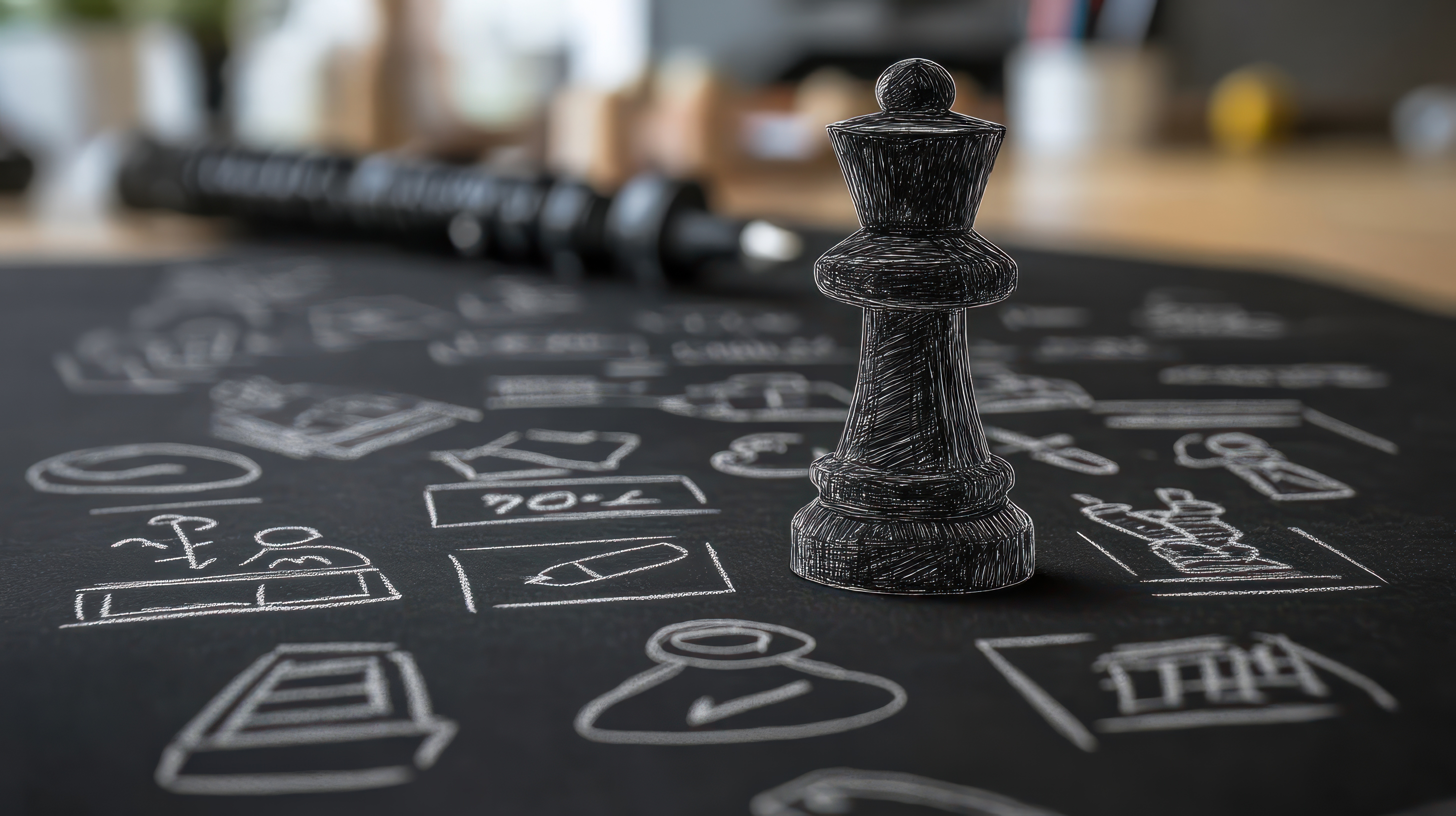
Let’s be real: most AI strategy decks are 50% buzzwords, 50% vaporware.
They show off slick copilots, talk about “transforming workflows,” and then hand you another dashboard. Another chatbot. Another pile of stale data with a fancy UI.
That’s not strategy. That’s stalling.
The teams actually winning with AI? They’re not building for show. They’re building for outcomes — the kind that compounds over time, not just once.
AI Is Not a Strategy. Outcomes Are.
Everyone wants to say they’re “using AI.” Very few can show what it’s actually doing for them. LLMs are powerful — but they’re not magic. Slapping GPT onto a workflow isn’t a strategy. It’s a shortcut.
Real AI strategy is about what gets done — what moves faster, what becomes smarter, what scales without dragging human teams down.
You need to ask simple, brutal questions:
- Is research faster?
- Are agents finding, collecting, and structuring new intelligence automatically — or are you still waiting on stale reports?
- Are insights sharper and more actionable?
- Does the system help you decide faster and better — or just summarize what’s already outdated?
- Is updating automatic and constant?
- Or does your intelligence degrade every day because your data is frozen in time?
If the answer is no across these points, you’re not building an AI system. You’re building a liability.
Most "AI Integrations" Are Lipstick
Too much AI today is surface-level. It’s a chatbot bolted onto an old workflow. It’s a "copilot" that still needs a human pilot every five minutes. It’s dashboards over stale, static data that doesn’t learn or adapt.
Real AI systems work under the surface.
They don’t just automate the front-end. They automate the work itself:
- Researching
- Structuring
- Validating
- Updating
Real agents don’t just react — they reason. If your "AI" can’t think through a task — if it can’t reason, adapt, and act — it’s lipstick. And lipstick doesn’t move the needle.
Design Systems That Execute, Not Just Impress
The future isn’t one genius agent. It’s systems that mirror how real teams operate:
- Specialized roles
- Coordinated execution
- Continuous improvement
You don’t win with one smart assistant. You win with purpose-built agents linked together into living, breathing workflows that can flex, grow, and get smarter over time.
Agents should be embedded wherever real work happens:
- New intelligence captured: agents that proactively surface fresh data — not wait for a prompt.
- Data validated and structured: not just scraping or summarizing, but applying rules, context, and quality checks autonomously.
- Insights refreshed continuously: the system needs to be live, not locked in a quarterly report cycle.
Behind the scenes, these systems are orchestrated — modular, agent-driven workflows tuned for speed, quality, and insight.
No duct-taped demos. No fragile chains. No one-trick bots. Real workflows. Real execution.
Good AI Strategies Build, Layer, and Learn
The strongest AI strategies don’t just automate tasks. They compound intelligence over time.
Winning teams design around three core principles:
1. Dynamic Data Products:
- Intelligence isn’t static. It’s a live product.
- Real systems don’t just "clean" data — they build structured, layered, living datasets that update, connect, and expand.
- Data products aren’t raw feeds. They’re full experiences ready for agents to use immediately.
2. Specialized Agents:
- Agents need to be domain-specific, task-specific, and outcome-driven.
- A research agent operates differently than a validation agent.
- Build modular teams of specialized agents that can coordinate, hand off tasks, and reason through workflows — just like human teams do.
3. Feedback Loops That Compound:The best AI systems aren’t static.Every execution feeds learning back into the system:Over time, intelligence accelerates — and your edge grows while competitors fall behind.
- New data refines models
- Model outputs tune agent behavior
- Fresh insights trigger new workflows
Real AI Systems Are Secure by Design
When you move from passive dashboards to active, autonomous agents, security isn’t optional. It’s fundamental.
Good AI strategies bake security into the system architecture, not bolt it on later. Key security considerations when working with agent-driven systems:
Data Governance at Every Layer:
- Agents must respect access controls, sensitivity labels, and data residency rules — automatically.
- If an agent pulls, transforms, or links information, it needs to enforce security policies as part of the workflow, not after the fact.
Controlled Autonomy:
- Agents must operate within clear boundaries.
- Not every agent should have full read/write access everywhere.
- Smart design limits what agents can access, how they act, and how outputs are surfaced.
Auditability and Traceability:
- Every agent action — from a research pull to a validation step to a decision assist — needs to be logged, timestamped, and reviewable.
- You don’t just need results. You need a trail.
Model and Data Isolation:
- In critical use cases, agents should avoid exposing sensitive data directly to external models (e.g., uncontrolled API calls).
- Keep proprietary data protected inside secure, managed workflows.
Security isn’t about slowing agents down. It’s about enabling trusted, autonomous execution — at scale. If your AI strategy ignores security, you’re not building an advantage. You’re building a liability.
Unbullsht your AI strategy
Build systems that ship real work, not slides.
Agents. Output. Intelligence that compounds.
That’s the game now.
Blog
Aenean lobortis, massa a imperdiet iaculis, lorem odio lacinia elit, non hendrerit ligula justo tempor lorem.